Case Study
The Next Generation of Pharmaceutical Development Using Self-Learning Models
£8,000 saved per development batch
Testing time reduced from months to minutes
The Challenge:
Predicting the dissolution kinetics of corticosteroid particles emitted from a nasal spray from the size and shape distributions of those particles. Nanopharm’s goal was to optimise a particle size/shape distribution from a target dissolution profile, in order to save development time. How quickly the corticosteroid particles dissolve relates to how quickly the product will get to work in the body after administration.
The Solution:
A random forest regression model was trained to predict the mass dissolved at different time points from the size and shape of the particles to a good degree of accuracy. The resulting Monolith dashboards enabled users to upload a target dissolution profile and return an optimised particle size and shape distribution that would produce that target dissolution profile.
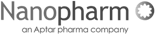
The Company:
Nanopharm is a cutting-edge pharmaceutical business – specializing in orally inhaled and nasal drug products (OINDPs), ultimately providing development services for pharmaceutical companies developing both new and generic drug products. Nanopharm operates with around 50 key employees, and is owned by Aptar who employs around 13000 people. Their target market is any size pharmaceutical company that is developing an inhaled or nasal drug product.
The Challenge
Predicting the rate at which particles delivered by a nasal spray dissolve
Nanopharm is the leading provider of orally inhaled and nasal drug product design and development services globally. In a recent project, Dr. William Ganley, Head of Computational Pharmaceutics, and his team adopted Monolith as an AI solution during the test phase of their expensive and time-consuming classical methods to predict particle dissolution rates.
Their leading goal was to optimise a particle size/shape distribution from a target dissolution profile, in order to save development time and costs. The standard approach to measure both the size/shape distributions and dissolution profiles took them around 3-6 months for large test campaigns. Predicting these results would lead to faster and cheaper tests.
Before using Monolith, Nanopharm tried to build mechanistic physics-based models to predict the dissolution kinetics of corticosteroid particles. This method took a day to measure six dissolution curves, and two more days to generate 12 replicates for a single batch which added up to several months of measuring and testing as other measurements were conducted in parallel. The classical approach fell short of delivering accurate results for highly insoluble and irregularly shaped particles.
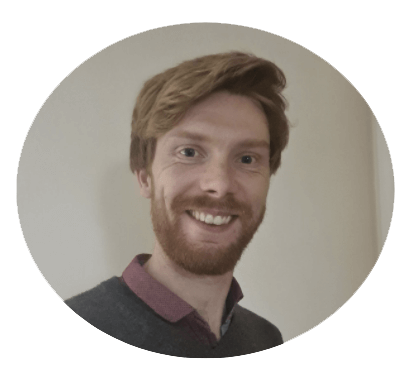
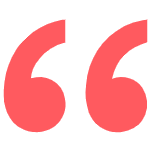
“These models showed poor predictive capability, we had a relatively small data set so did not know whether a machine learning approach would be possible.”
- Dr. William Ganley, Head of Computational Pharmaceutics at Nanopharm Ltd
The Approach
Approaching the problem with an AI partner
Nanopharm wanted to know whether pharmaceutical lab-scale datasets were sufficient to employ a self-learning model approach to quickly understand and instantly predict the performance of the complex chemical system, but also have guidance and support from ML subject matter experts at Monolith.
Will and his team first heard about Monolith from colleagues in Aptar that had benefited from using the low-code AI platform, and attributed success to their partnership. Nanopharm decided to piggyback their program, and soon adopted Monolith as a solution to reduce their overall testing & development time.
Nanopharm wanted to use AI to build a physics agnostic predictive model. More simplistically, they did not want to use a mechanistic model as they had tried before, and instead went with a purely data-driven approach with no information provided about the underlying physics or chemistry, coupled with a methodology that could be applied to other types of pharmaceutical lab data. The ability to optimise time-consuming measurements like particle size/shape from faster cheaper measurements like dissolution, was key to reducing development time and costs for their customers.
Nanopharm used Monolith’s self-learning model capabilities to develop a data processing and modelling method. Nanopharm was able to design workflows that facilitated modelling and optimisation of the required size/shape distributions, cut testing times from two days to less than two minutes for a single batch, and saved around £8,000 per development batch. Additionally, it allowed them to develop performant customer-specific models.
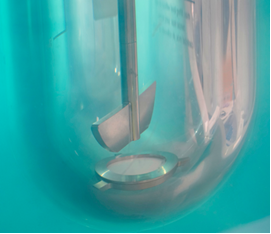
A paddle over disk dissolution apparatus used in this study
Project Workflow
Data pre-processing - The advanced join method
The idea for the project workflow was data pre-processing. This included summary statistics of the size distribution, and deciding whether or not to include time as an input feature for the dissolution, followed by model screening, model validation, template notebook, and dashboard generation. The Monolith features used were summary statistics from the size and shape distribution data, such as means and standard deviations of particle diameter, convexity, perimeter, circularity, etc.
One challenge that arose during the project was around data quantity. The team’s data sets were relatively small, and the particle size and dissolution data had a different number of rows (25 per batch in particle size and 12 per batch in dissolution). The team at Monolith quickly stepped in, and advised Nanopharm to screen a number of different machine learning algorithms such as linear regression, Gaussian process regression, random forest regression, neural network, and regression models and see which performed better for the size of data set they had.
The Monolith experts came up with an ‘advanced join idea’, where the rows from the larger data set were joined to random rows from the smaller data set (within each batch) to get the largest number of data points from all the available data. This method repeated rows but ensured all data was used. Following this ‘advanced join’, data related to two batches were removed and saved for use to validate the performance of the model, while the remaining data of the other four batches was used to train the models. Will’s team then checked the performance of models built using the validation data from the two ‘unseen’ batches.
Results
Accurate results & AI platform praise
To begin with, Monolith reduced the number of inputs because the dataset was not big enough. To decide which of the size/shape measurements were important, Monolith’s data science team suggested adding a bit of custom code to generate the summary metrics, taking the mean standard deviation and other metrics.
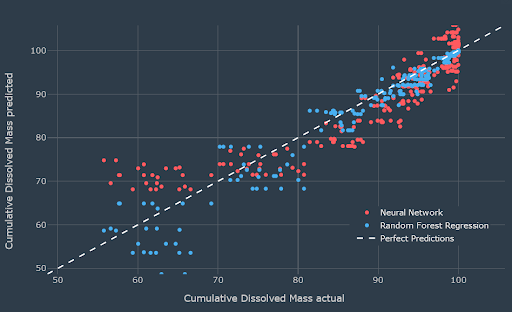
Actual vs. predicted cumulative dissolved mass for the test set showing the good predictions of the random forest model
A random sampling method was then suggested to bring the MDRS (Morphologically Directed Raman Spectroscopy) and dissolution data together in a way that maximised the data points. Monolith guided Nanopharm on how to put these results into a dashboard so that it would be easily understood by others, and to turn a very technical and statistical project into a user-friendly dashboard.
The final model predicted the dissolution data to a good degree of accuracy. The results obtained were predicted dissolution time curves from particle size and shape data, and optimised particle size/shape data from target dissolution profiles. Predictions of dissolution kinetics at short time points (<5 mins) were slightly under-predicted. The team had 84 particle size/shape measurements and 72 dissolution measurements. Particle size/shape distribution measurements take around 6-8 hours, and they can run 6 dissolution measurements in 3 hours which is 30 minutes per run - all of this time effort could be reduced using the self-learning approach using Monolith.
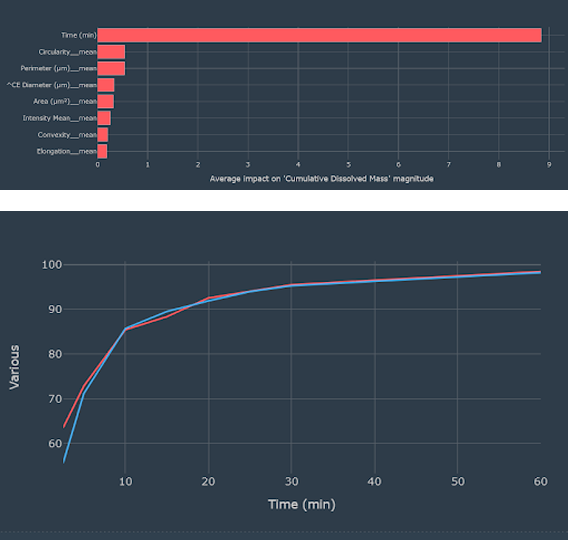
Feature importance showing that particle shape parameters are important in predicting dissolution in addition to the time at which the dissolution was sampled
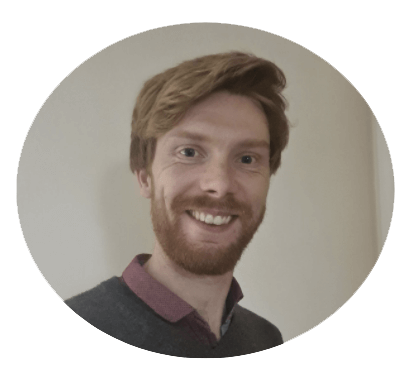
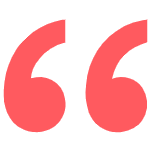
“Nanopharm’s Computational Pharmaceutics group is always on the lookout for new ways to save laboratory or clinical time using computational solutions. This collaboration with Monolith fits right into our vision for the future where many stages of the pharmaceutical development process can be expedited or even replaced by fast computational methods.”
- Dr. William Ganley, Head of Computational Pharmaceutics at Nanopharm Ltd
Next Steps
Next steps for Nanopharm & Monolith: continued collaboration
Since adopting Monolith, the solution they have developed has real potential to shorten the development time for their customers. Will’s team can now also benefit from a template method for building additional similar models with different types of data.
With a trusted AI partner, Nanopharm can see a future where the time-consuming laboratory methods can be predicted from the quicker and more cost-effective alternatives, allowing them to screen larger numbers of designs quickly, and only proceed to the time-consuming measurements with the promising candidates. In the future, they would like to extend the methodology to other measurement types and market this solution as a service to their customers.
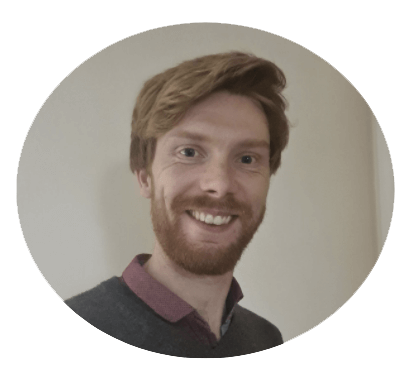
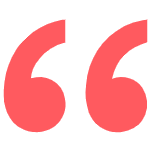
“Monolith seems to be able to weave AI solutions to solve any problem using any data set.”
- Dr. William Ganley, Head of Computational Pharmaceutics at Nanopharm Ltd