Meet Our AI Experts at Professional MotorSport World Expo
See How AI Is Used by Automotive Engineers
Booth: 2064
Köln Messe, Cologne, Germany
November 9, 10, 11, 2022
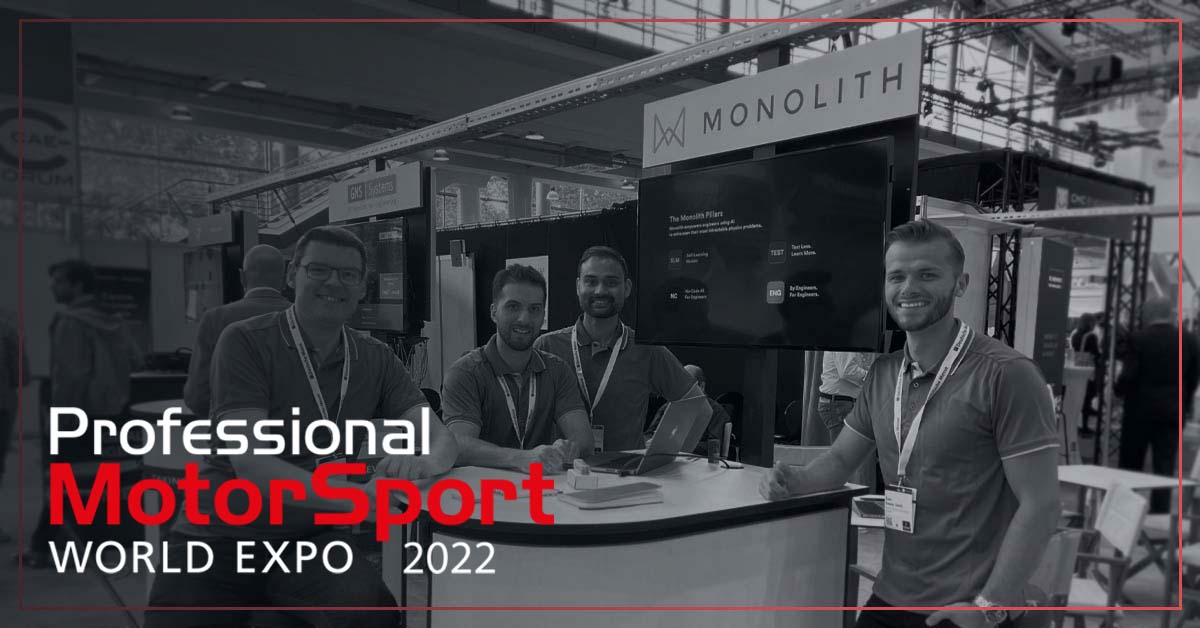
Technical Presentation
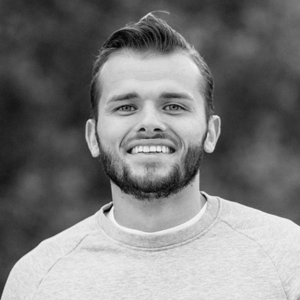
Self-Learning Models for Vehicle Dynamics
Learn how to use AI to solve your most intractable physics problems. This presentation will focus on track dynamics, using a customer case study in collaboration with Jota Sport, who decreased testing time by ~72% using Monolith’s intelligent exploration tools. Discover how Machine Learning (ML) models can be trained on specific manoeuvers to predict the forces on the vehicle during maneuvers that were not performed, thereby reducing total testing time.
Trusted by

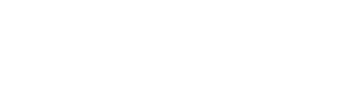
About Professional MotorSport World Expo:
Professional MotorSport World Expo returns this November for three days, showcasing the latest and best technologies for motorsport and ultra-high-performance road car applications! Following an enforced hiatus, the 2021 show’s popularity proved the continued importance of being able to meet fellow industry professionals in person and exchange ideas.
Motorsport is undergoing a technological shift, with sustainable powertrains seeing rapid adoption and across the board there is ever greater access to advanced technology at all levels of racing.
About Monolith:
We enable engineers all over the world to:
- Understand physically intractable problems
- Fully explore multiple virtual test scenarios
- Reduce costs and time investment throughout the whole R&D cycle
- Increase confidence in predictions & recommendations on which tests to run next
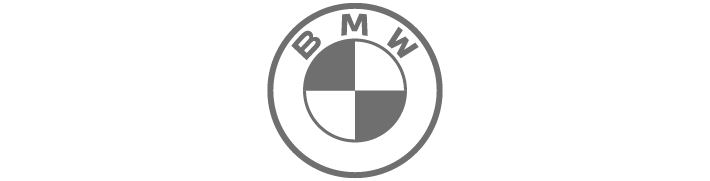
"Using Monolith, BMW Group engineers built self-learning models using the wealth of their existing crash data and were able to accurately predict the force on the tibia for a range of different crash types without doing physical crashes."
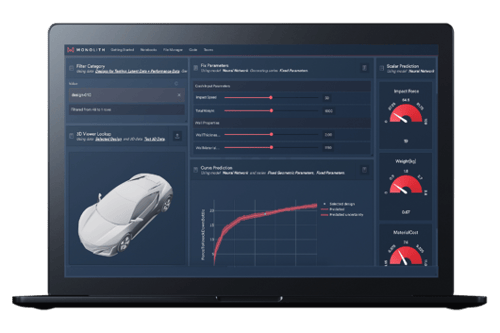
Popular Monolith Resources
Automotive White Paper, Webinar, and Case Study
Case Study
Jota Sport Cuts Car Setup Time by 50% With Monolith AI
Since teaming up with Monolith AI, Jota engineers can better understand and predict the aerodynamics of their cars by building self-learning models. As a result, they have reduced the number of simulations and tests by 50%, cut car time-to-setup in half, and achieved a 66% reduction in overall costs.