Monolith
Reduce battery test time with AI.
Using data-driven AI models, you can better understand and predict battery performance to significantly reduce testing time without compromising coverage or safety.
Why Monolith?
Machine Learning to guide your engineering decisions.
Batteries drive the cost, performance, and complexity of EVs. Don't risk falling behind with a traditional testing approach. Optimise your test plans with AI to slash time-to-market.
✔️ Accurately model and predict battery performance
✔️ Reduce battery validation from months to weeks
✔️ Shorten product development duration significantly
Ensure battery design quality & safety using AI
With the power of AI, you can model battery performance across the design space with a fraction of a traditional test plan.
Using the Next Test Recommender, you can apply multiple machine learning algorithms at once to chart your testing path using the fewest steps possible incrementally.
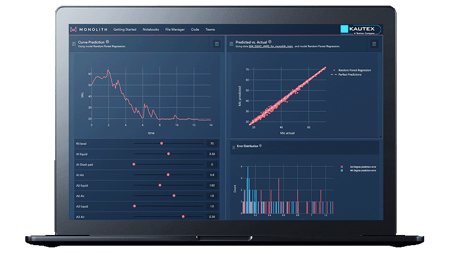
Predict the critical tests to run
Test too much and you waste time confirming what you already know. Test too little and you risk missing performance issues. Schedule, quality, and your career depend on finding the balance.
- Run the most important tests and skip the rest
- Optimize resources spent on costly test rigs and facilities
- Validate your designs faster with fewer prototype iterations
"Using Monolith AI platform, we were able to import our rich test stand data and apply machine learning models to conclude on different development options much faster."
Dr. Bas Kastelein, Sr. Director of Product Innovation, Honeywell Process Solutions
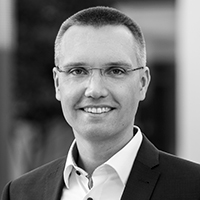
Predict Next Tests to Run Using Machine Learning
Monolith AI introduces the Next Test Recommender (NTR)
- Engineers can now access ranked validation test recommendations, reducing testing by 30-60% and speeding up time to market
- With human-in-the-loop active learning powered by AI, domain experts can optimize test pans for complex products in automotive, aerospace, and industrial domains
A commissioned study conducted by Forrester Consulting on behalf of Monolith
The State of AI in Engineering
The first-ever study on AI in product development surveys US and European automotive, aerospace, and industrial engineering leaders.
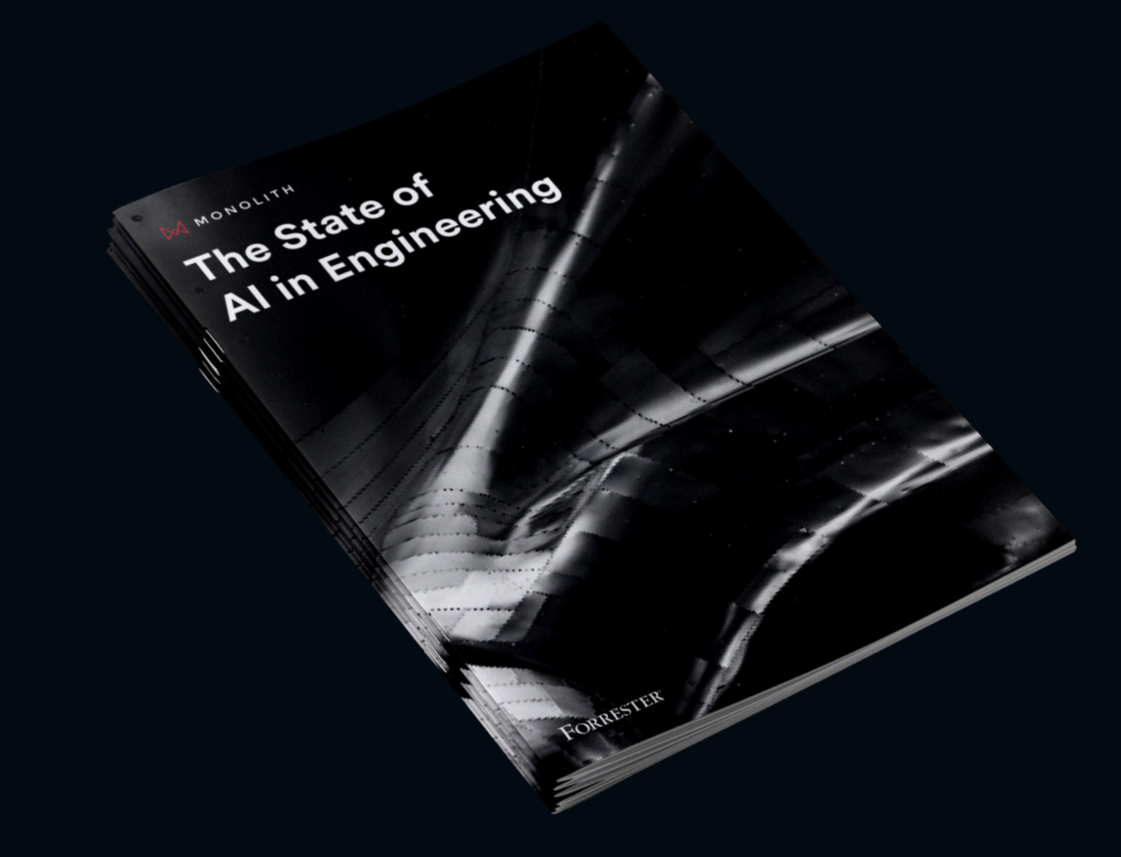
"If your model is in your data, Monolith will find it. Built by engineers for engineers, Monolith helps you make better models faster."
Dr. Ted Duclos, Monolith Advisor and Former CTO at Freudenberg Sealing Technologies
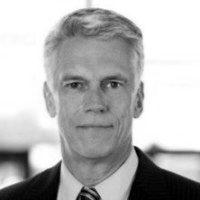
No-Code AI Software
By engineers. For engineers.
- Avoid wasted tests due to faulty data
- Build just the right test plan - no more, no less
- Understand what drives product performance and failure
- Calibrate non-linear systems for any condition
Battery testing FAQs
My engineers are busy. Why risk trying Monolith over another AI tool?
Monolith is the only AI platform built by engineers for engineers and trusted by the world’s top engineering teams including BMW, Honeywell and BAE. Its advanced capabilities analyse complex engineering data sets quickly and accurately to help you find relationships, new insights and anomalies so you can trust your data fast. With its user-friendly, no-code interface, domain experts can quickly get up to speed and leverage institutional knowledge for future generations.
How is Monolith better than current statistical and simulation methods?
There are five ways Monolith delivers superior performance to statistical and simulation methods.
- Increased accuracy: advanced machine learning algorithms identify complex patterns and relationships in engineering datasets for more accurate predictions and insights
- Faster analysis: algorithms are optimised for speed, allowing it to analyse large datasets much more quickly
- More comprehensive analysis: analyse both structured and unstructured data
- Adaptive learning: continually refine and improve the accuracy of predictions
- Ease of use: no code environment built by engineers for engineers
What if I don't have alot of data or I'm modeling a new product?
Most often, you need less data than you think! 100s of rows and a handful of columns for a tabular data approach can already get you going on your journey to adopt AI. If you don't have enough data for an AI project, we recommend the following:
- Data augmentation: Create new training examples from existing data by applying transformations or scaling to existing data (this is how our customer Kautex Textron did it).
- Transfer learning: Use and reuse data gathered from an old product to improve a new product.
- Consider a simpler model: A simpler model requires less data to train. The best approach will depend on the specific task and constraints of your project.
Our mantra: Start small - but start! Find a small, incremental quick win, and let our AI team of experts guide you on your way forward to intelligently acquire more data and build even more impactful models.
How will having a model in Monolith help me reduce design cycles?
Monolith reduces design cycles by offering rapid analysis, accurate predictions, custom models, collaboration, and optimization capabilities. Its algorithms are optimized for speed, enabling quick iteration and testing of multiple scenarios. Custom models capture domain-specific knowledge, while collaboration and optimization capabilities improve effectiveness and identify the best design options. By leveraging Monolith's capabilities, teams can deliver better designs more efficiently.
Does my team need to start from scratch once an AI model is built?
Monolith's self-learning models automate repetitive or time-consuming tasks, allowing teams to focus on more complex work. However, data quality, model drift, and interpretation are critical considerations, all supported by Monolith. Teams may need to update or retrain models to account for changes and understand their decision-making processes. By monitoring and managing these factors, teams can ensure ongoing value from Monolith's AI models.
Learn more about Monolith
Recommended Resources
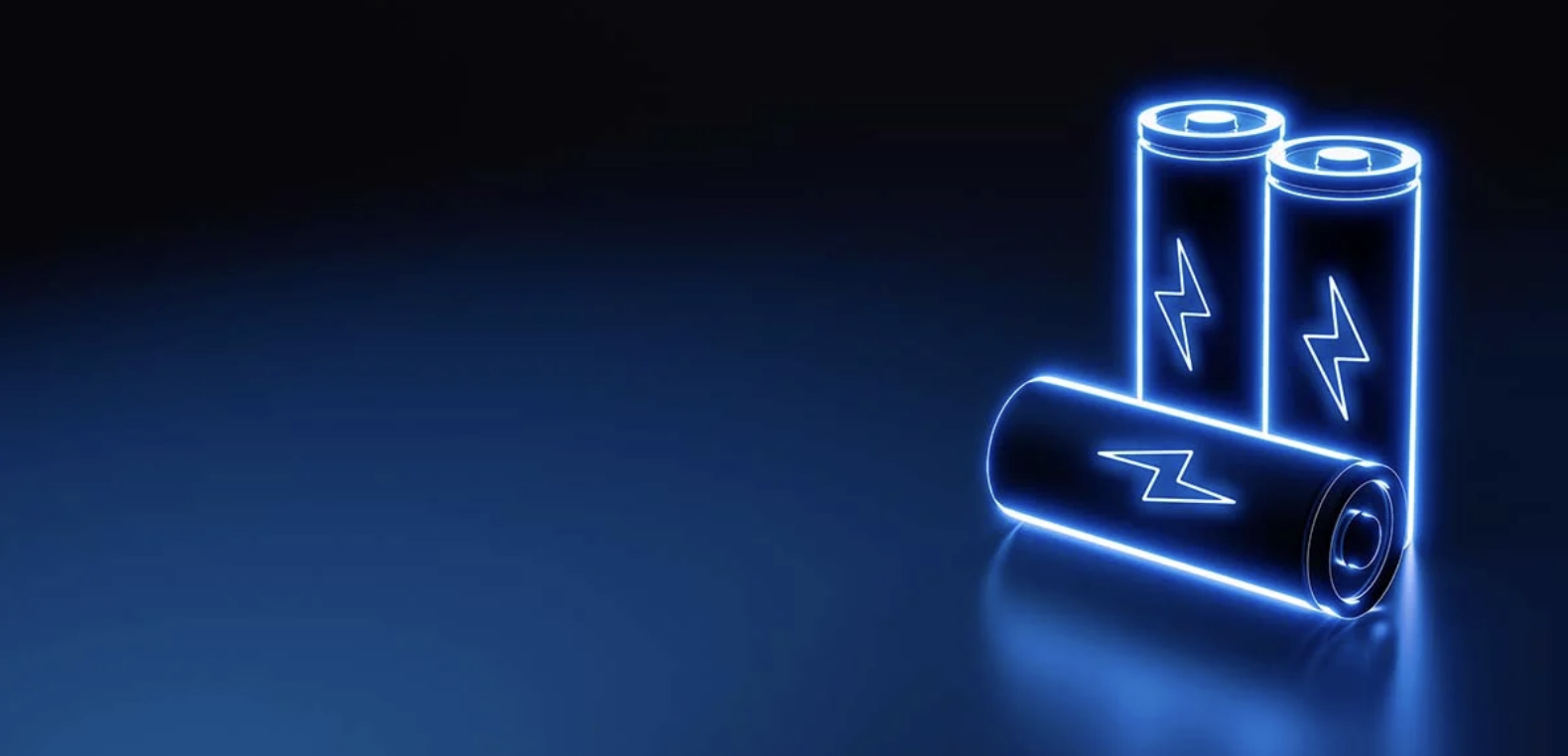
Challenges and Opportunities in Fuel Cell Testing
AI Battery Fuel Cell Testing: Optimising Performance Efficiency
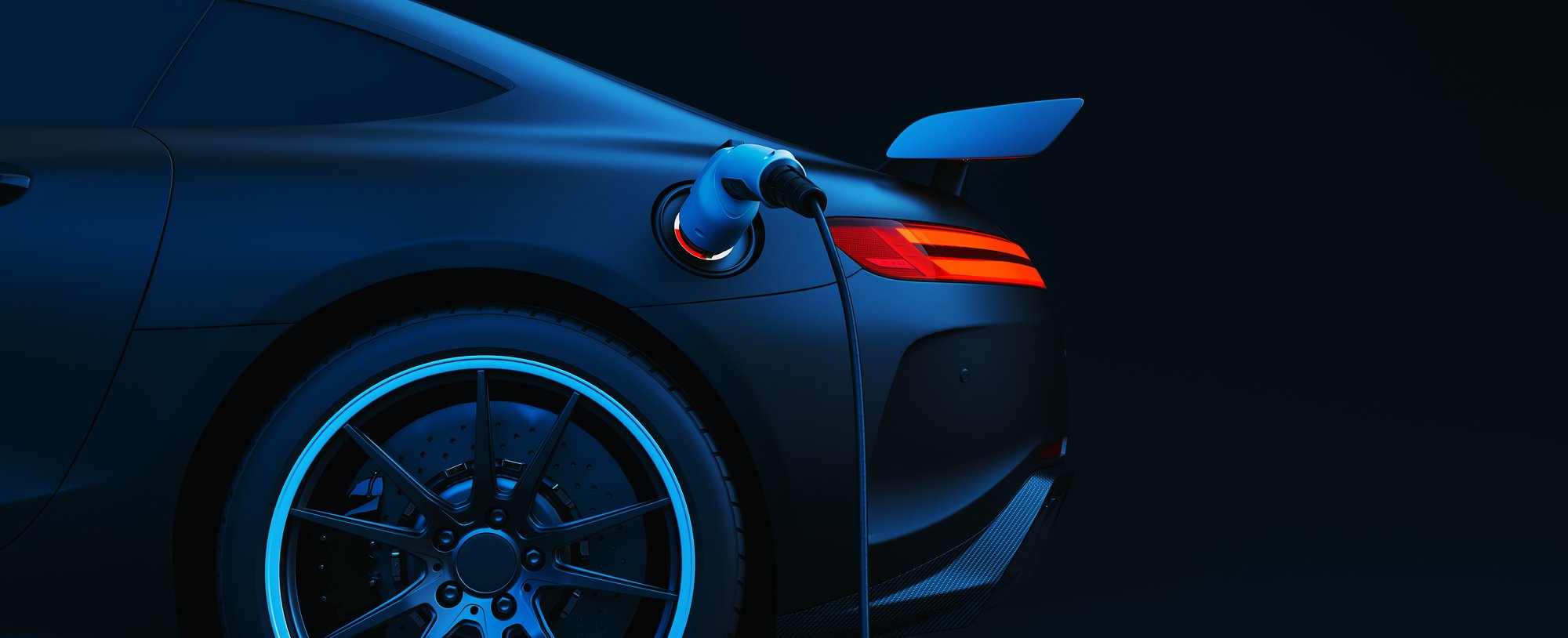