Among the multiple applications of machine learning for engineering and product development, we have recently improved our optimisation capabilities, with a focus on categorical optimisation.
Categorical variables are widely used in engineering and often, the design phase requires selecting the optimal value of a category (e.g. material type, manufacturing process, …).To do so, we collaborated with the prestigious university of ISAE-Supaéro (Toulouse) to develop new methods of optimisation combining both continuous and categorical variables.
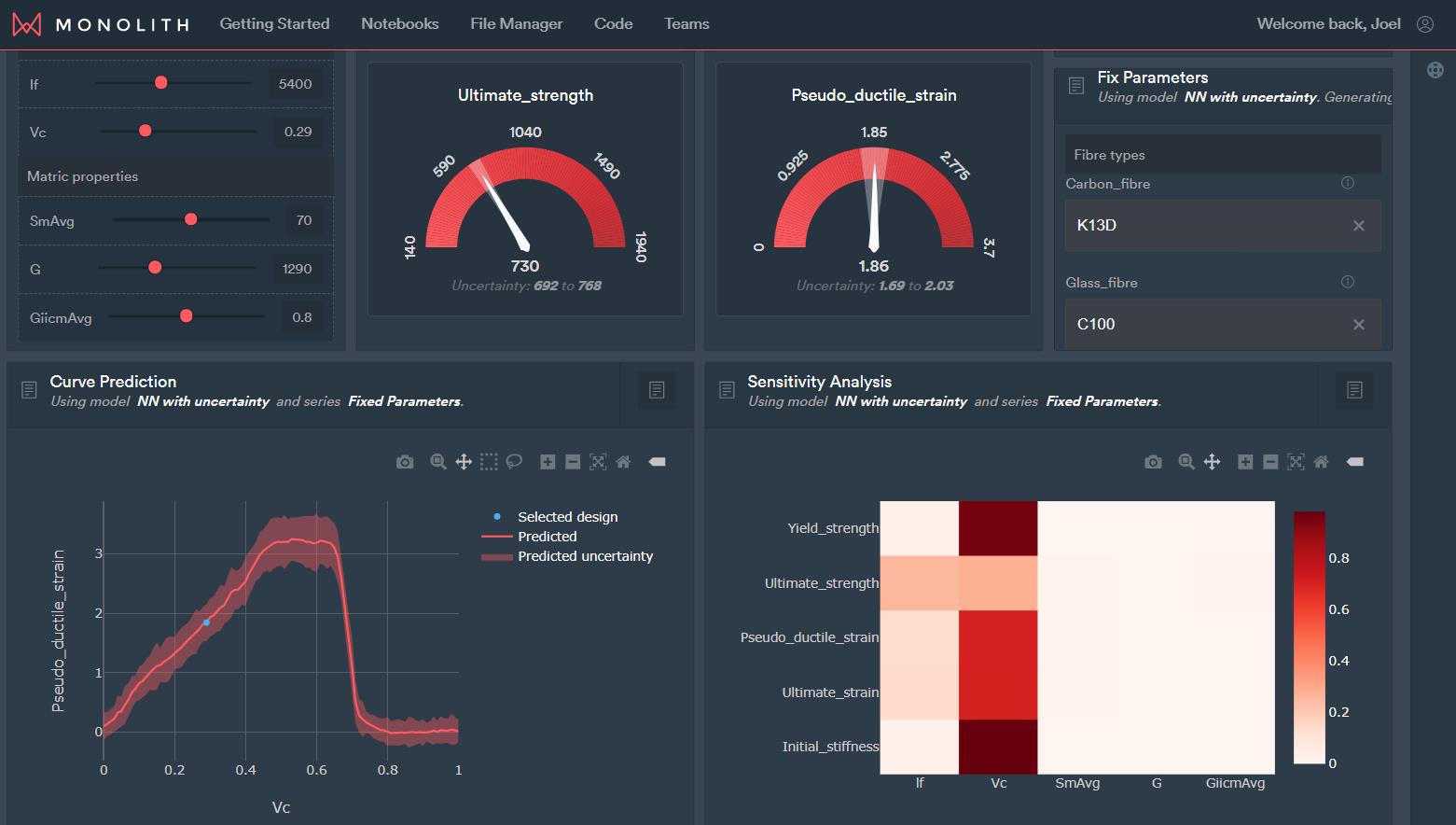
Surrogate models are an essential engineering tool and their popularity has increased recently due to the high computational cost of evaluating real-world simulations.
However, most of these functions are described by mixed variables (continuous and categorical), which makes it harder to create accurate interpolation functions. This work builds a surrogate model from a given mixed data set, in order to quickly and accurately calculate the mechanical performance of hybrid discontinuous composites.
Then, in order to find the optimal hybridization, three different approaches are performed: mono-objective, inversion and multi-objective. Starting from an experimental database, the mixed categorical-continuous optimization process is performed by coupling a multi-armed bandit strategy and a continuous Bayesian optimization algorithm. The efficiency of our proposed approach is tested and two main results are achieved.
Firstly, the obtained surrogate models are shown to be sufficiently accurate, having an R2 score grater than 90% in average.
Secondly, our proposed optimization process is able to identify correctly optimal fibres with respect to desirable targets provided by the industrial partner.
Contact us if you would like to know more about this topic.