Among the multiple applications of machine learning for engineering and product development, we have recently used the monolith platform to perform what we call “virtual testing”. Test campaigns can be expensive, but also time consuming. And sometimes, after all tests are completed, you might realise that you would have liked to make more tests. That is where virtual testing can help. The concept is to train machine learning models on existing test data and use the trained model to predict the outcome of other – virtual – test campaigns.
This has multiple applications. The two main ones are that you can perform:
- A virtual campaign of a test that you could do but that you have not done yet, so that you can save time and money
- A virtual campaign of tests that you cannot do for the moment, so that you can have insight of what the behaviour would be in this situation.
The two applications above will be now illustrated by looking at the calibration of an engine, for which a test campaign was already performed. The data collected during these tests contain the operating conditions of the engine (engine speed and torque), sensor values measuring properties such as the temperature, pressure, … within the engine, and overall outputs like the brake-specific fuel consumption (BSFC), which was the output we focused on in this study.
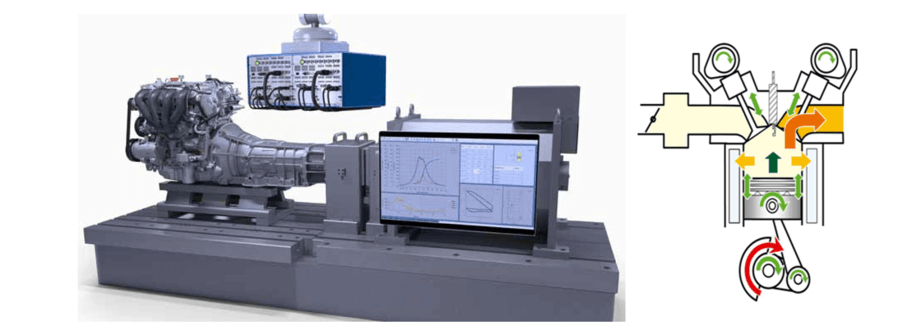
Left: engine test bench. Right: Engine cut section
For the first application, the objective was to find out what would be the effect of starting the combustion cycle slightly earlier than in the test campaign (1 degree earlier). After training a model, this model was used to virtually re-run all the tests from the first campaign, but with a combustion starting 1 degree earlier, and predict the fuel consumption. Shell graphs were then used to understand which area of the operating conditions could benefit from this change. In this case, it appeared that in regions with low engine torque and high engine speed, fuel consumption could be reduced by around 2%.
For the second application, the objective was to quantify the effect of reducing the charging loss (lower friction when exiting the chamber). Research could be performed to try to reduce the charging loss by 5%,but that would be really costly (changing coating, valve timing, …), and therefore it is important to make sure that it is worth doing it. This time, the model re-run virtually all the tests by reducing the charging loss by 5%and estimated the new fuel consumption. Shell graphs showed that this had little impact for low engine speed (below 2000 rpm). However, for higher speed, the fuel could be reduced by 0.5 to 0.7%, which could make it worth it.
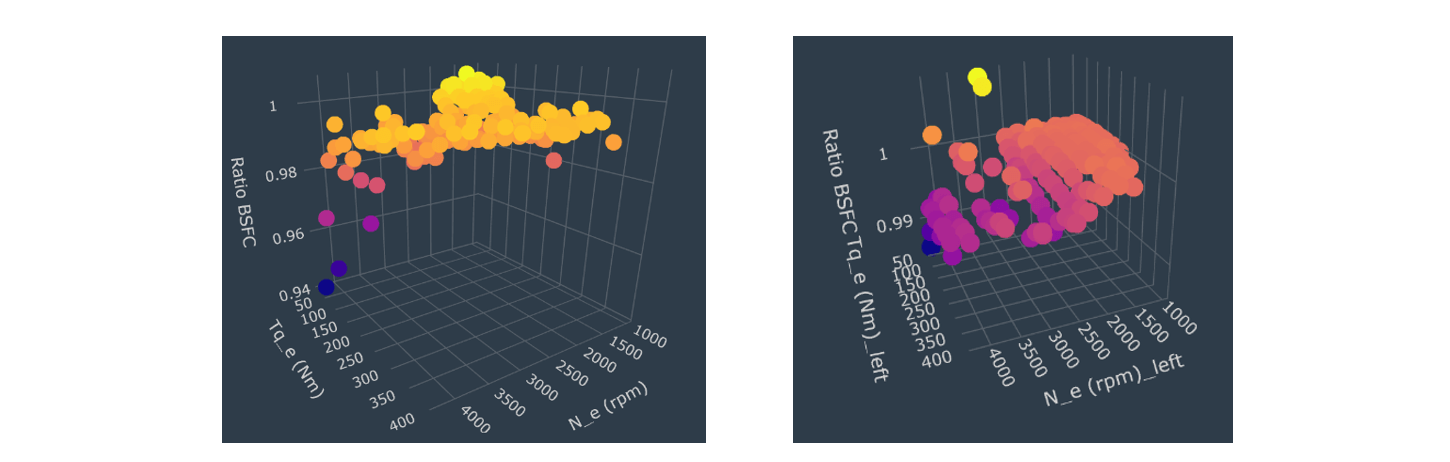
Left: impact of earlier combustion on BSFC. Right: Impact of reducing the charging loss by 5% on BSFC.
As you can see, both applications have different benefits. The first one enables to predict results that could be collected, but that would require time and money, thus delaying the time to market of the new product. The second application enables to virtually test something that could not be tested otherwise. Here, you gain insights that you could not have at all. You get a snapshot of the future to make better decisions in the present.
For both applications, the key advantage here is that although engineers might guess the expected trend (e.g., less friction yields lower fuel consumption), such virtual test campaigns will add a missing yet critical information, which is a quantitative value. Now the engineer knows that her product can consume 2%, or 0.7% less fuel, and she can quickly make appropriate decisions.